Recent mass shootings in Buffalo, Uvalde, Tulsa, and more have left scores dead from sickening gun violence in just the last few weeks. The wave of injuries and deaths has revived the political debate surrounding America’s uncommonly lax gun laws, raising hopes for reform. Yet we’ve seen cycles of this sort before, with the hopes of victims, their families, and their communities dashed by congressional inaction, with even mild responses like universal background checks extinguished by the constitutionally built-in ease with which minorities can block action in the Senate.
Real reform is not impossible. The 1994 assault weapons ban passed by large margins in both House and Senate, also in the wake of a deadly school shooting. But to carry out such reform, Congress will have to overcome two key factors: the NRA’s entrenched position within the Republican Party, and the ability of the NRA and other interest groups to dismantle the current bicameral Democrat majority by peeling off legislators who take gun lobby money.
The NRA’s ties to the Republicans are well understood, but the second is much less publicized. It turns out that votes on gun control legislation can be predicted with 95% accuracy when using legislators’ campaign finance receipts from the pro-/anti-gun lobbies and the gun industry, with the effect of campaign money statistically significant. In particular, a Democrat who takes any amount of NRA money is about 4 times more likely to cast an anti-gun control vote as those who don’t. The conclusion is inescapable that the gun lobby is powerful not just because it funds nearly every Republican politician, but also because it targets individual Democrats whose votes can be bought or rewarded.
Too many guns
As of this writing, more than 200 mass shootings (defined as 4 or more people shot in each such event) have occurred in America in the first half of 2022. Many such events feature shooters who obtained their weapons with nauseating ease. The Uvalde, Texas shooter—18 years of age—used a website to purchase the semi-automatic assault rifle he used to massacre 19 children, picking it up eight days before his rampage. The Tulsa, Oklahoma shooter bought a handgun from a pawn shop and, three days later, an AR-15. The same day that he bought the AR-15, he shot up a hospital.
The supply of guns in America is enough to arm each adult, child, and infant in America with some 50 million guns to spare. We have twice as many guns per person as the runner-up: Yemen. To be sure, part of the reason is cultural. Fully 40% of households have a gun. An American adult is more likely to live in a household with a gun than they are to have a bachelor’s degree. This cultural attachment to the gun is sharply partisan, to such an extent that the absolutist defense of the right to bear arms has become a core interest of the GOP.
In the context of very small partisan margins in the House and Senate, this has led to partisan gridlock on gun legislation, despite the fact that substantial aspects of gun reform are not controversial. Even when moderated by sustained political debate with massive campaign spending on both sides, voters polled in statewide referenda have delivered enhanced background checks with an 86% success rate this century.[1] Yet, federally, even mild national-level reforms like closing background check loopholes are systematically killed in the Senate.
A new analysis
In a representative democracy, the baseline expectation for policy design should be the majoritarian position: policies that the majority of voters prefer should determine legislative outcomes as a default. Deviations from that state of affairs are what have to be explained. A prima facie plausible explanation is the role of campaign contributors. Elections are expensive, and our campaign finance laws are permissive. To meet the costs of running, politicians accept campaign contributions from those with the means to provide them, which tends to mean the wealthiest. When those contributors are particularly well organized, they can channel those investments into access, votes, and influence.
My recent INET working paper showed that legislators’ individual votes can be predicted with high accuracy using Political Action Committee (PAC) contributions data, and that including such data always led to better predictions than party-level baselines. That analysis,[2] which is based on statistical summaries of campaign finance data and an inventory of about 400,000 individual votes, allows us to demonstrate this effect at scale, but does not differentiate between votes in different policy domains, and does not allow us to link predictive effects back to particular donors or interest groups (see the endnotes and Fig. 6 for discussion).
Here, I narrow the scope by limiting the analysis to gun control measures, including as predictors only PAC contributions from three categories: pro-gun control and anti-gun control ideological PACs, and gun industry PACs (vendors and manufacturers), using PAC category annotations provided by Opensecrets.org. As discussed below, PACS are a subset of all contributions, but the starkness of the evidence here suggests that they are likely reliable as a rough indicator of preferences.
Method. I examined 14 votes—7 in the House and 7 in the Senate—identified as key national gun policy votes by VoteSmart. Every item in VoteSmart’s list was included if it was primarily about gun policy[3] and could be unambiguously interpreted as pro- or anti-gun control.[4] To get numerical summaries of bill content, official summaries of each bill/amendment assembled from congress.gov were embedded using a neural network[5] and then projected into 5-dimensional text feature vectors using principal component analysis. The merits of this feature generation approach to text data are discussed in (Lalisse 2022).
The final dataset[6] includes five bills/amendments strengthening background checks, one that weakened them, a semiautomatic assault weapons ban, a ban on high-capacity magazines, two bills nationalizing state-level concealed carry laws via interstate reciprocity, a bill guaranteeing the right of “mentally incompetent” veterans to buy a gun, and a pair of bills blocking certain gun laws in the District of Columbia—including the “Defend Our Capital Act of 2015”, which would have legalized concealed carry for visitors to the District of Columbia, and might in retrospect have been called the “Make Insurrection Deadlier Act.”
The bills were manually assessed for directionality (pro-/anti-gun control) by inspection. For the main analysis, the roll calls (legislator-by-legislator voting records) were retrieved from the VoteView research database. The resulting table of votes (N=3720) was split into 10 cross-validation partitions, holding out 10% of legislators at each fold. The baseline is the party-line vote: a held-out legislator votes how the majority of their party voted in the training fold. The main predictors of interest are the summed contributions to a legislator from each of 22 gun-related PACs from 2008 to 2020.[7] If gun money is informative over and above party affiliation, we predict higher accuracy for models including the gun PAC features, relative to the baselines.
Results. Table 1 displays results for an array of models combining the following predictive features:
- GUNFIN: 22 d vector summarizing the legislator’s contributions from gun-related PACs (one feature per PAC)
- LEGFIN: 25 d vector summarizing the legislator’s campaign receipts from all PACs (PCA applied to all institutional donors, see Lalisse 2022).
- LEGPRTY: 3d indicator for the legislator’s party
- SPONPRTY: 3d indicator for the party of the bill/amendment sponsor
- BILLVEC: a 5d vector representing the bill/amendment, based on a neural network embedding of the measure’s text summary
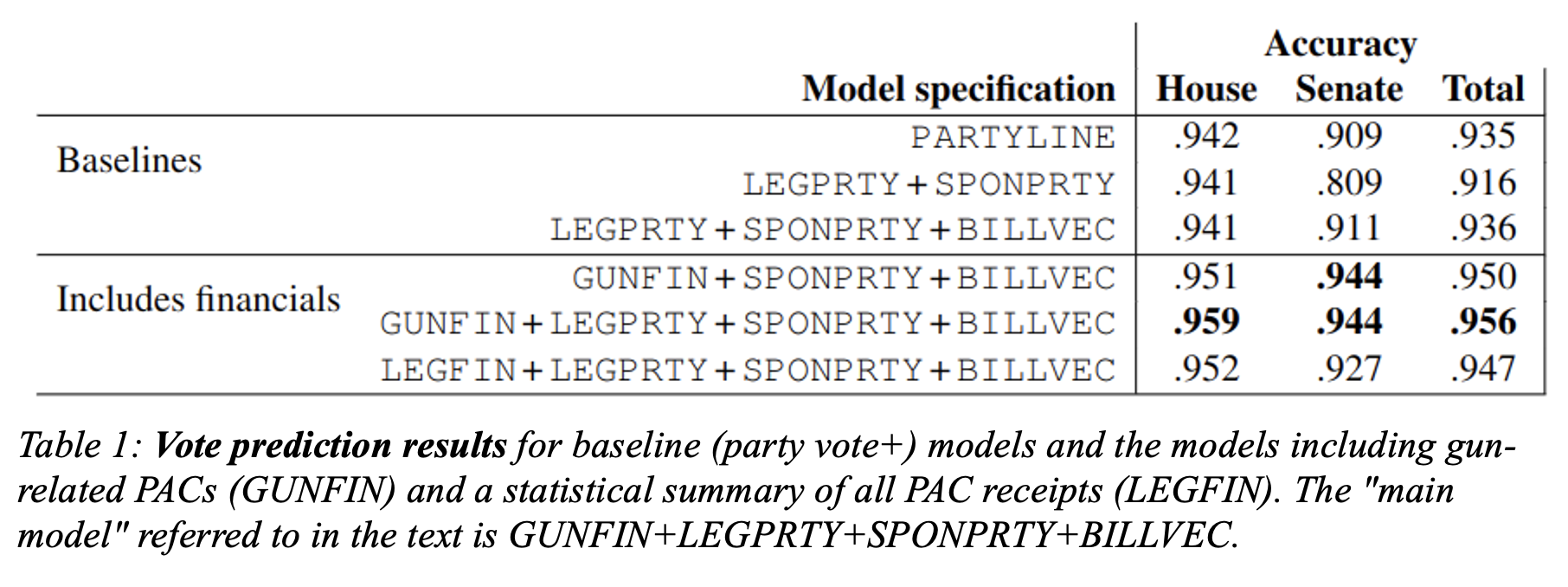
The main model performed best, with a 3.5% improvement in the Senate and a 1.7% improvement in the House, which amounts to 3 additional Senate votes and about 7 additional House votes predicted per roll call, over and above the party-line vote. This result is significant at the ɑ=.001 level, as confirmed by a permutation-based protocol.[8] Furthermore, GUNFIN outperforms LEGFIN (the all-PAC summary vector), which itself outperforms the baselines, validating the earlier approach while also reinforcing the hypothesis-driven approach taken in the current analysis.
Assessing directionality. GUNFIN groups together information from three broadly-defined interest groups:[9] (a) pro-gun ideological groups, (b) anti-gun ideological groups, and (c) the gun business (e.g. Smith & Wesson). This typology is misleading. Yes, it is surely the case that there is no organized industrial interest backing the anti-gun movement.[10] Congressional campaign spending by organized interest groups seeking gun regulation is basically a new phenomenon.[11] Anti-gun spending was negligible until the 2014 election cycle, when Gabby Giffords—following the tragedies of Sandy Hook and her own attempted assassination—formed Americans for Responsible Solutions as a counterweight to the NRA (see Fig. 2). Direct campaign spending by gun industry actors does not show up in most conventional tabulations of campaign spending, which do not analyze individual contributions by executives (see Ferguson et. al. 2020 for discussion, and solutions, in an analysis of the banking sector).[12]
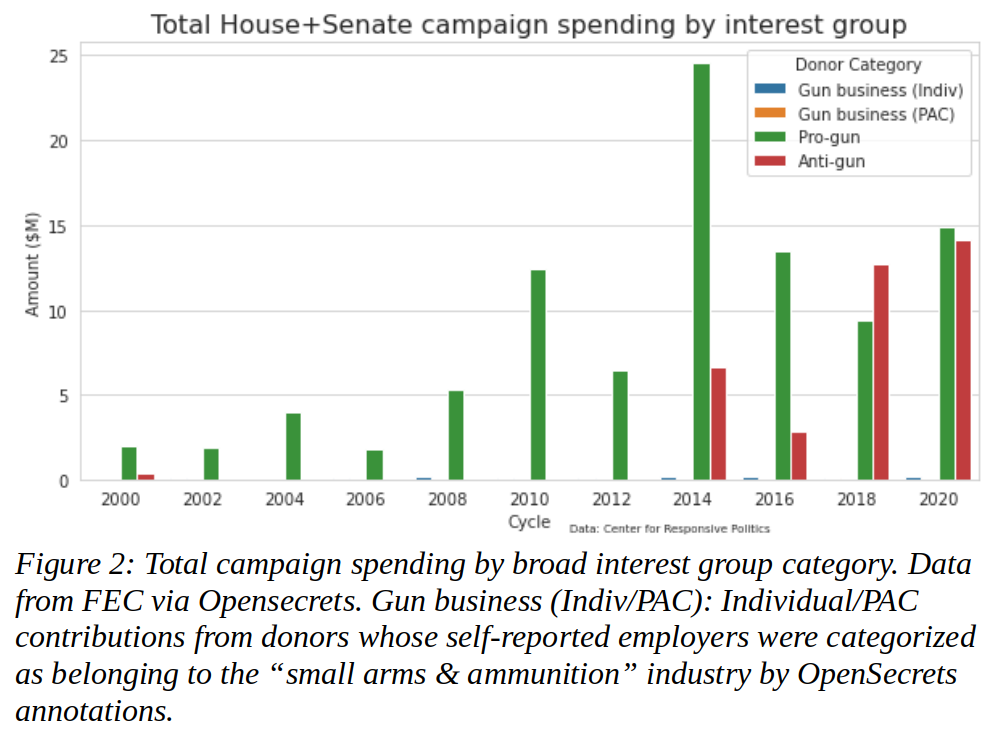
However, to view the NRA as an “ideological PAC” in contrast to gun business PACs is surely not correct. The NRA brands itself as a grassroots civil rights organization, but its political activities would be impossible in the absence of backing from the gun industry, whether via direct contributions or through the vast network of weapon vendors and manufacturers that staff and sponsor NRA events, without which a politically mobilized consumer culture oriented around the gun is fairly hard to imagine. It is thus more appropriate to say that the gun lobby operates as a semi-autonomous cultural organization whose primary purpose is to amplify the interests of its industrial base by transforming that base into an ideology.
These caveats aside, the predictions for each category are clear: pro-gun (“ideological”) and gun industry PACs should predict anti-gun control votes, and anti-gun (“ideological”) contributions should predict votes in favor of more gun control, particularly when crossing party lines (pro-gun to Democrat, anti-gun to Republican), with the following subtlety: that above-average contributions to a legislator within the interest group-aligned party (e.g. large NRA contributions to Republicans) may in fact be directed at legislators who need incentives to remain in the fold. This is essentially the relationship we observe (Fig. 3).
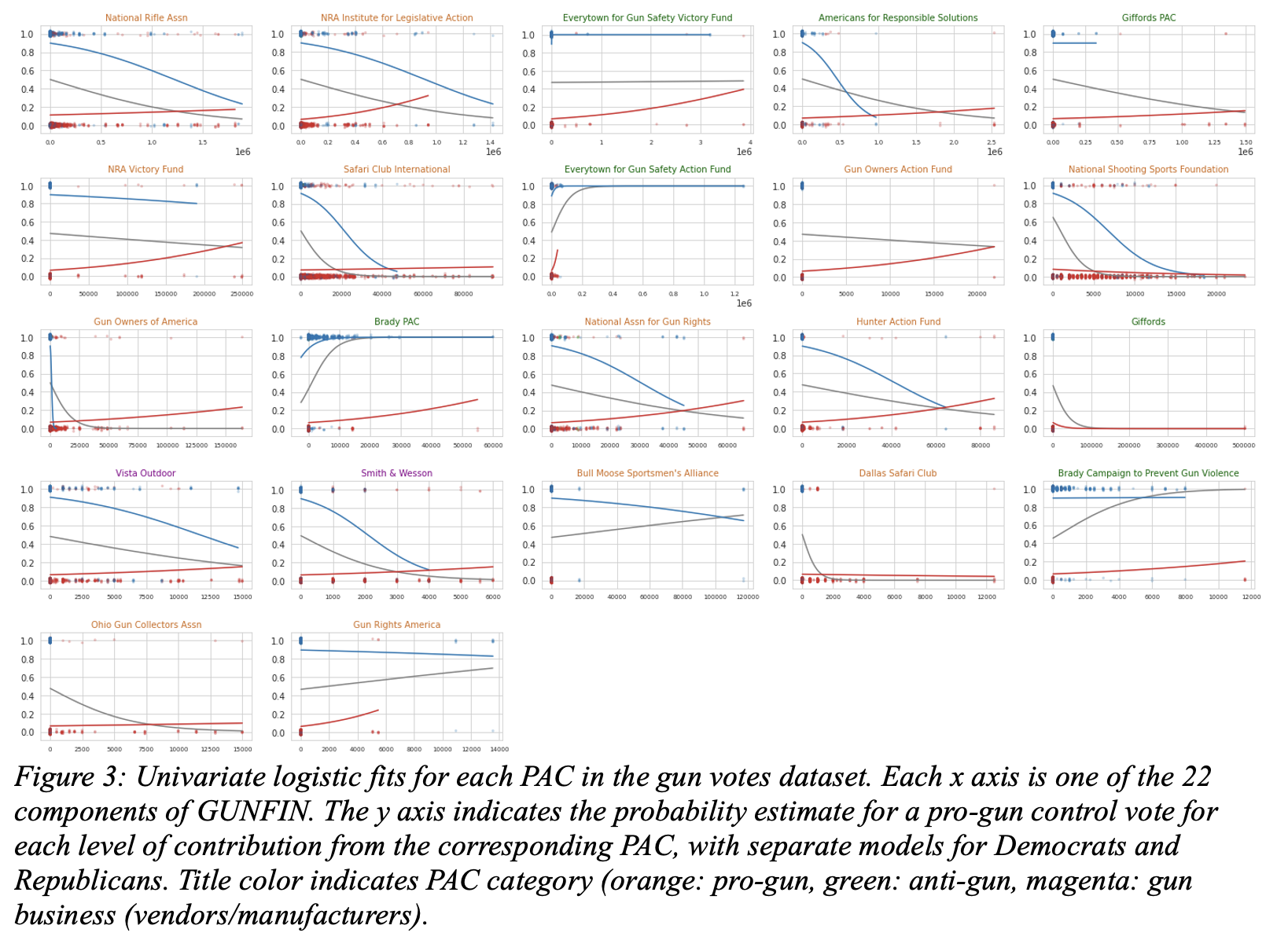
The additional predictive power of campaign finance in relation to legislators’ votes is significant both statistically and also in terms of its practical effects. Part of the reason the NRA holds sway in D.C. has to do with its influence on the Republican Party. That influence flows through contributions to party PACs, the campaigns of party leaders (GOP whip Steve Scalise is the largest individual recipient), as well as recurring contributions, spread so widely through the party that the interest group becomes a sustainer for most members (only 2% (11) of the Republican politicians in the gun votes dataset did not receive any NRA money).
An interest group thus embedded in the party apparatus can determine legislative priorities, affect the leadership’s commitment to whipping votes, influence the selection of party-endorsed candidates, and steer the party PACs’ contribution profiles (which candidates the party supports, and by how much). This sort of party control wielded by the NRA explains events like NY Congressman Chris Jacobs’ recent single-issue purging from the GOP after he endorsed an assault weapons ban—expulsion not by the voters, but by the party.
The broad architecture of this aspect of political money was described by Thomas Ferguson’s Golden Rule: parties are not vote-maximizers, but rather the manifestation of “blocs of major investors who coalesce to advance candidates representing their interests.” In line with this account, we are able to basically swap campaign finance data for the party and obtain better predictions than those we get from the party-line vote. GUNFIN is an effective proxy for party.
But it is also more than a proxy for the party. The true impact of targeted campaign finance is felt when a vote is within the penumbra of a key threshold (usually the 50% majority, but sometimes the 3/5ths filibuster threshold), in which case a sufficiently agile interest group with a bag of campaign recipients can influence those clients in the preferred direction.
The balance of power between the parties in recent Congresses has been increasingly narrow. The current climate of intense partisanship and a narrow party division are very much to the strategic advantage of businesses and other interest groups seeking to determine policy outcomes, since it means being able to count on partisan gridlock as the default scenario, with individual votes tilted through targeted mobilization of legislative assets. Without campaign finance reform that curbs maneuvers of this sort, policy-making on guns and other issues may well turn on such dynamics.
Money matters
We are all familiar with the following routine. A journalist reports on a large contribution from some interest group at odds with a politician’s stated values and seeks comment from the recipient. The cookie-cutter response given by a Henry Cuellar staffer—his boss caught red-handed with a $6,950 check from the NRA—may as well stand in for them all: “Contributions of any size have never and will never influence his policy positions.”
Who can say what was in Henry Cuellar’s heart when he voted to federalize Texan gun law? Or when he voted that “mentally incompetent” veterans who’ve had run-ins with the law—like the Thousand Oaks shooter—should be able to buy guns unimpeded? When he voted twice to rob D.C. voters of their will regarding the proliferation of guns on their streets? Or when he voted, after Uvalde and unlike every other Texas Democrat, not to ban high-capacity ammo clips that can be used to kill ten at a time?
Who can say? Yet, the following calculation may prove useful as an instrument to any voter faced with the choice between two politicians, each avowed to be clean of heart, with one taking NRA money and one who does not. In my sample, a vote from a Democrat who has taken any amount of NRA money (N=219) is about 4 times more likely to be a pro-gun vote. A vote from a Democrat who took $10,000 or more in NRA money (N=69) is 10 times more likely to be pro-gun than one from a Democrat who took none (N=189).
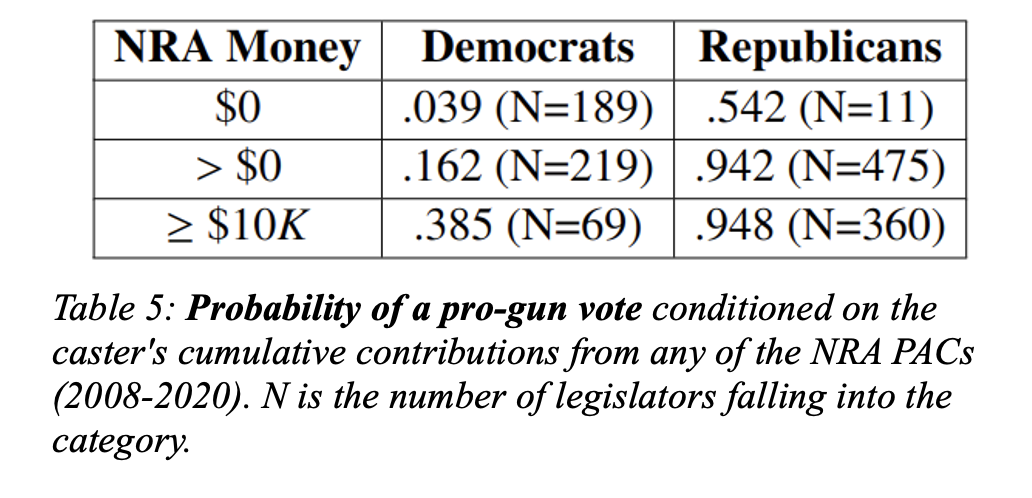
The facts speak plainly: if you want to know how your congressional representative will vote, look beyond the party label and follow the money.
Notes
[1] The National Conference of State Legislators’ ballot measure database records 7 such referenda since 2000—one each in California (2016), Colorado (2000), Maine (2016), Nevada (2016), and Oregon (2000), and two in Washington (2014 and 2018)—with Maine’s the only one that did not obtain a majority.
[2] The analysis in Lalisse 2022 did not differentiate between votes in different policy domains, and it also made use of statistical techniques that summarize highly correlated donation profiles into aggregate features (the output of principal component analysis) that do not easily trace back to individual contributors’ impact. The method also suffers from unmeasured directionality: a vote of “Yea” on a bill that is about “gun control” depends not just on the topic of the bill, but also whether the bill is pro- or anti-gun control (among other factors). Machine learning models of the sort used within this paradigm are able to estimate nonlinear decision boundaries that take into account interactions between features, e.g. by solving analogs to the XOR problem (see Fig. 6)—and indeed, models like this are unavoidable in order to capture such appropriately messy generalizations as “a Republican will never vote for an assault weapons ban, especially if that Republican takes NRA money and received their endorsement, except if that Republican is independently wealthy and represents an area recently afflicted by a mass shooting,” a generalization that would account for GOP Congressman Chris Jacobs’ recent change-of-stance on assault weapons. An analysis of this sort, which does not reduce to cumulative linear effects, can be captured in the coefficients of an appropriate nonlinear model. The resulting complexity, though, means that directionality becomes hard to assess.
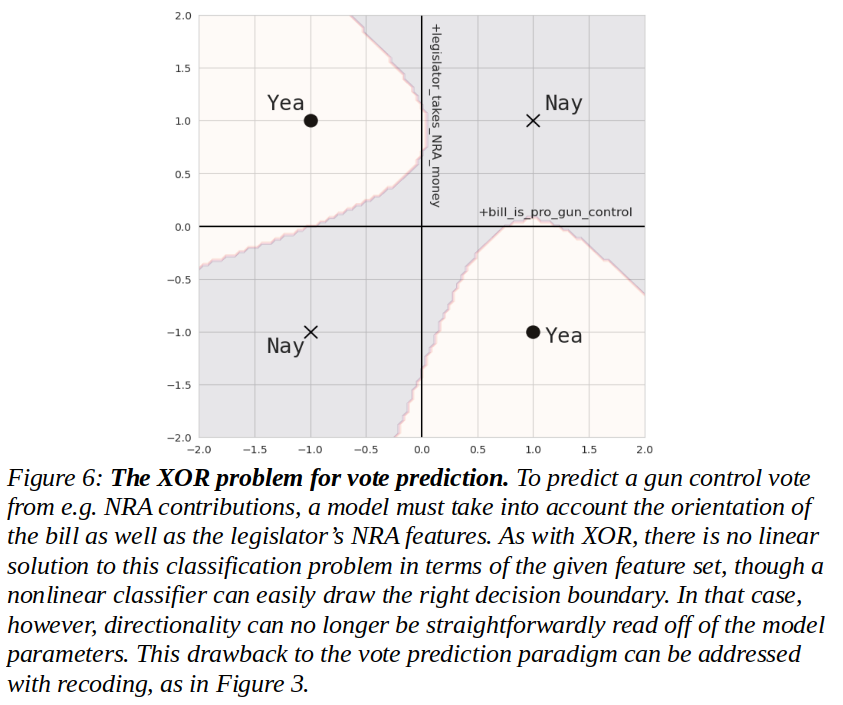
[3] Excluding, for instance, the March 2021 reauthorization of the Violence Against Women Act, a 311-page bill that included restrictions on gun ownership for those convicted of stalking or intimate partner violence alongside dozens of other significant measures unrelated to gun control.
[4] This included a pair of House and Senate amendments directed at gun laws in the District of Columbia, as there was clear directionality to those measures—e.g. the Defend Our Capital Act (S Amdt 2915), which would have enforced reciprocity of state concealed carry laws specifically within D.C., mirroring national-level reciprocity proposals. The clear directionality criterion excluded a series of 2016 Senate amendments imposing varying degrees of restrictions on gun purchases by suspected terrorists, as well as a bill making federal lands available for hunting.
[5] Beltagy, I., Peters, M., & Cohan, A.. (2020). Longformer: The Long-Document Transformer.
[6] The final sample includes 6 House bills 1 amendment. All 7 Senate votes were on amendments (the Senate has not held a vote on a standalone gun control bill since 2005, when it voted bipartisanly to immunize gun manufacturers from prosecution for shootings using their products). 2 cloture votes (votes to end a filibuster) were included, with votes of “Yea” interpreted as being in favor of the underlying measure.
[7] All gun-related PACs were included if they contributed at least $50,000 in the target period.
[8] In this procedure, each legislator was randomly reassigned to another legislator’s GUNFIN vector, with the model then re-trained and evaluated with shuffled campaign finance profiles. This was repeated 1,000 times. Parameters of the permutation distribution: μ(accuracy)=.927 , σ(accuracy)=.002.
[9] For this analysis, we use the typology developed by Opensecrets.org.
[10] Though it is also true that the gun reform movement is woven in with billionaire Michael Bloomberg’s ambitions to solidify his stance within the political establishment.
[11] See Fig. 2, though see also Opensecrets’s review for historical spending on lobbying by anti-gun groups, which is more substantial, but still dwarfed by pro-gun spending.
[12] The financial data used in this study is limited in many respects, though I believe PAC data has some advantages also. While they can be straightforwardly and accurately classified into broad interest group categories (with the sorts of caveats mentioned in the text), they only account for about a quarter to a third of direct campaign contributions, while individual contributions data are famously incomplete in the FEC listings. Grouping together contributions from individuals with the same employer requires labor-intensive scripting for employer name normalization and various other operations. Furthermore, the mapping from individual to employers is sometimes one-to-many. Take Elon Musk, (former) CEO of companies in financial services, space, biotech, automotive, batteries, highway tunnel infrastructure, and a variety of other industries. Other forms of money are typically left out of most tabulations. See the analysis in Ferguson et. al. 2020 for discussion, and solutions, in an analysis of the banking sector.