Daniel McDonald is Assistant Professor of Statistics at Indiana University. His research interests involve the estimation and quantification of prediction risk, especially developing methods for evaluating the predictive abilities of complex dependent data. This includes the application of statistical learning techniques to time series prediction problems in the context of economic forcasting, as well as investigations of cross-validation and the bootstrap for risk estimation. Daniel did his undergraduate studies at Indiana University where he received two bachelors degrees: a Bachelor of Science in Music with a concentration in cello performance from the Jacobs School of Music and a Bachelor of Arts in economics and mathematics. Prior to graduate school, he worked as a Research Associate at the Federal Reserve Bank of St. Louis. He received his Ph.D. from Carnegie Mellon University in statistics where he was named graduate student of the year for 2012 and received the Umesh Gavasakar Memorial Thesis Award for his dissertation “Generalization Error Bounds for State Space Models”.
Daniel McDonald
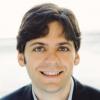
By this expert
Time Series Forecasting: Model Evaluation and Selection Using Nonparametric Risk Bounds
We derive generalization error bounds — bounds on the expected inaccuracy of the predictions — for traditional time series forecasting models.